Temporal adaptation aids object recognition in deep convolutional neural networks in suboptimal viewing scenario's
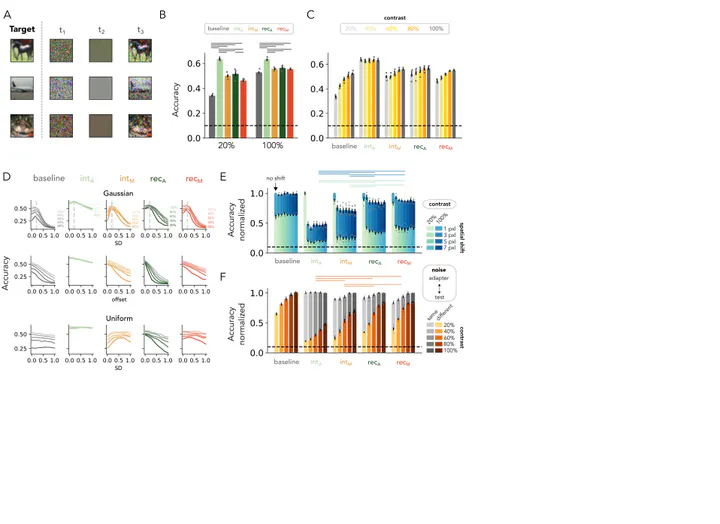
Abstract
The primate visual system excels in recognizing objects under challenging viewing scenario’s. A neural mechanism that is thought to play a key role in this ability is rapid temporal adaptation, or the adjustment of neurons’ activity based on recent history. To understand how temporal adaptation may support object recognition, previous work has incorporated a variety of temporal feedback mechanisms in deep convolutional neural networks (DCNN) and explored how these mechanisms affect object recognition performance. While multiple adaptation mechanisms have been shown to impact model behavior, it remains unclear how the origin (intrinsic or recurrent) and the way the temporal feedback is integrated (additive or multiplicative) affects object recognition. Here, we compare the impact of four different temporal adaptation mechanisms on object recognition using three different task designs, including object recognition under either noise or occlusion, and in the context of novelty detection. Our results show that the effectiveness of temporal adaptation mechanisms for robust object recognition depends on the task and dataset. For objects embedded in noise, intrinsic adaptation excels with simple, high-contrast inputs, while recurrent mechanisms perform better with complex, low-contrast inputs, highlighting their focus on different visual features. Under dynamic occlusion, recurrent adaptation mechanisms exhibit a more progressive increase in performance over time, suggesting they better maintain object coherence when parts are obscured. For novelty detection, recurrent mechanisms show higher performance compared to intrinsic adaptation mechanisms, suggesting that recurrence aids in detecting global changes caused by the presentation of new objects. All together, these findings suggest that robust object recognition likely requires multiple temporal adaptation strategies in parallel to handle the diverse challenges of naturalistic visual settings.
Type
Publication
BioRxiv Preprint